Curious about how transphobia finds its way into the algorithms that we encounter everyday? We got you covered! Kelsey Campbell gave a talk at the 2021 Responsible Machine Learning conference, introducing gender diversity, cissexism in automated systems, and leading research in the field!
Check out the recording online and learn why we should, and how we can, do better to capture the complexity of gender in our data systems and be more inclusive!
While you’re at it, enjoy an entire playlist of Gayta Science educational talks on our YouTube channel! Don’t forget to “like” and “subscribe”!
Transcript for the recorded talk below!
Introduction
Hey everyone! Thank you so much for joining today and a huge thank you for having me at this conference on responsible machine learning! I am Kelsey Campbell and I will be doing a presentation on cissexism or transphobia in automated systems, which is an under-discussed aspect of the already very under-discussed field of responsible machine learning.
As a quick introduction – I am a data scientist in industry, I have worked in public health research, software development, and government consulting. I am also the founder of Gayta Science, which is a site devoted to highlighting the LGBTQ+ experience using data science and analytics! We have been around a few years now, and have a growing team of data folks, developers, traditional researchers, and designers who are all really passionate about the intersection of data and LGBTQ+ issues. We have published a variety of data journalism pieces, from super fun pop culture-y type things to serious, discrimination and injustice focused pieces. So please check us out online, and follow on the socials, there are a lot of good things there!
So the main thing I want to talk about today, is that gender is COMPLEX! It’s a deeply personal, individual thing that is completely non-apparent, non-obvious, and honestly just extremely confusing! It can be confusing for someone to understand their own gender, the gender of others, the concept of gender as a social construct in general. Whether recognized or not, gender is truly a beautiful and complex, undefinable thing.
Unfortunately, we live in a society that has taught us that gender and sex is binary, the opposite of complex. We even see it being taken a step further these days with automated gender classification. The idea that a computer (or a largely homogenous group of computer programmers) would be accurate in determining someone’s gender, when the reality is that gender is hard for HUMANS to understand is completely unrealistic, absurd, and in a lot of ways dangerous.
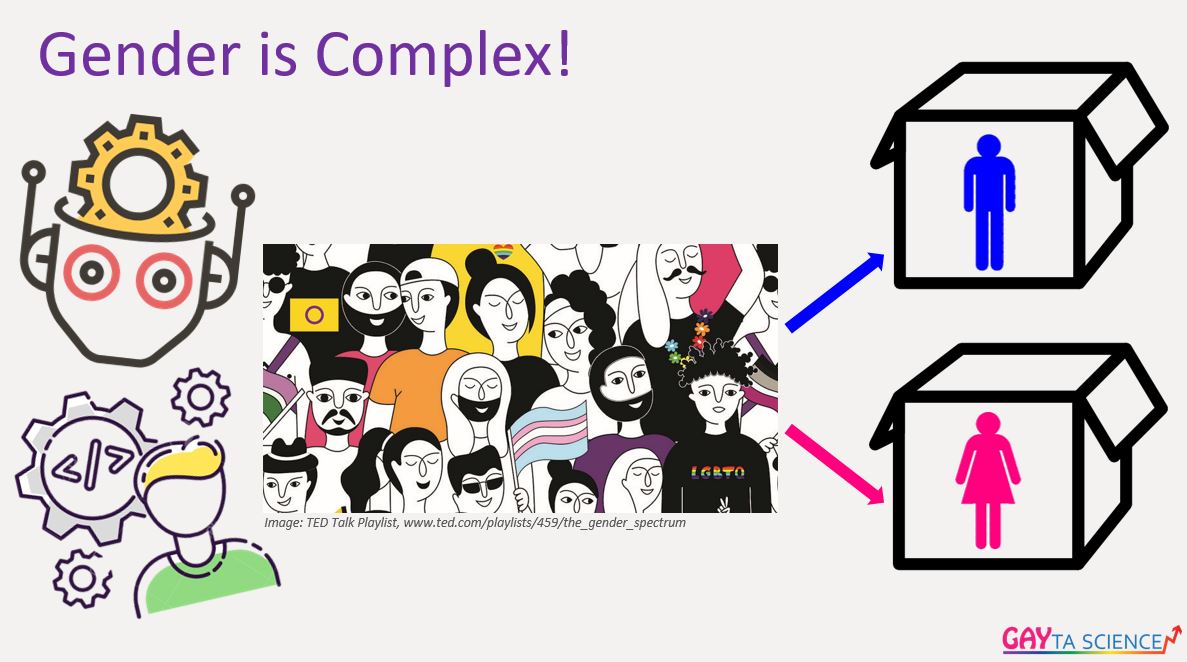
So I am a data person, and I’m aware that ALL data that we use is a simplification of reality in some way, but as more information and understanding is available, and younger generations are more aware and vocal about gender beyond the binary, our systems need to evolve. These rigid “Male” and “Female” boxes just aren’t working for people.
- Some people are going to move from one box to another
- Some people are in both
- Some don’t fit in either
- Some are in the middle
- Some are going to move around
There is just so much more to gender than our society has taught to recognize, and we can do so much better than binary data systems.
So today's talk is about why we should and how we can do better to capture the complexity of gender in our data systems and be more inclusive. I think these pictures do a great job of illustrating this – they are from Britchida, an extremely talented queer abstract artist that I encourage you to check out!
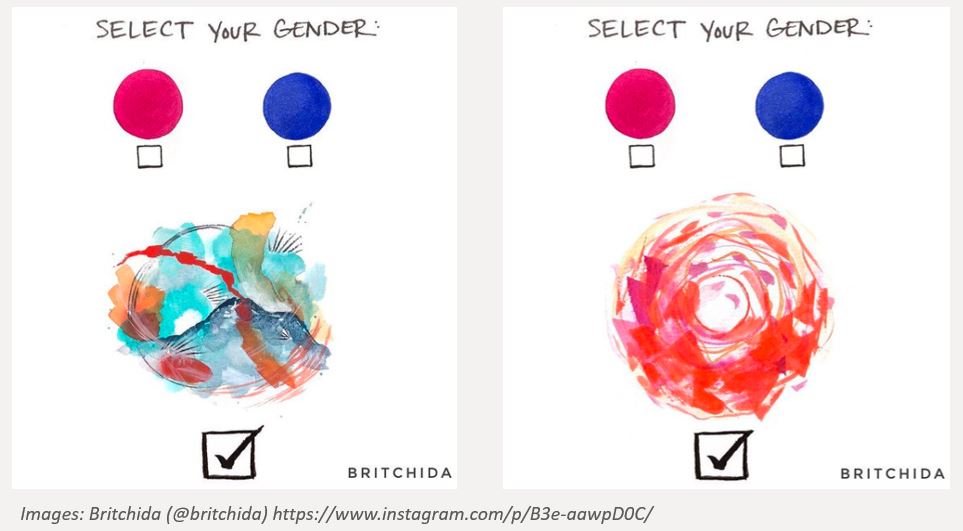
We will have a 4 part presentation today:
- Starting with a quick Gender 101 – maybe a review for some folks, but I just want to make sure that everyone is aware of the terminology I will be using.
- Then I will discuss some examples of Cissexism in Machine learning or automated data systems and what this looks like in real life.
- Followed by some of the current research in this intersection, which will not comprehensive by any means, but I want to highlight the main players in the space and hopefully show you some interesting things to check out if you are not already tracking.
- Then I will end with some actionable things that we can all do to do better in this space.
And as part of this, if we have time, I would love to share a VERY EARLY draft of a tool I’ve been brainstorming that would hopefully help with an aspect of doing better in this field.
But that is the overall plan for today! Let's jump right in!
Gender 101
So, like I said before, gender is COMPLEX - but the easiest way that I have found to conceptualize it is to use my friend the gender unicorn! The gender unicorn breaks down and illustrates different dimensions of identity, showing that gender identity (or your internal sense of being a man or a woman or something else) is different than your gender expression (how you “perform gender” or present yourself to the world through your clothes, hair, voice, body, mannerisms, and so on), which is also separate from your sex assigned at birth (typically what a doctor classified you as after looking at your genitals or chromosomes). The gender unicorn also includes dimensions for sexual and romantic orientations, showing that those are separate from gender and can be different. There are many different combinations of all these different dimensions.
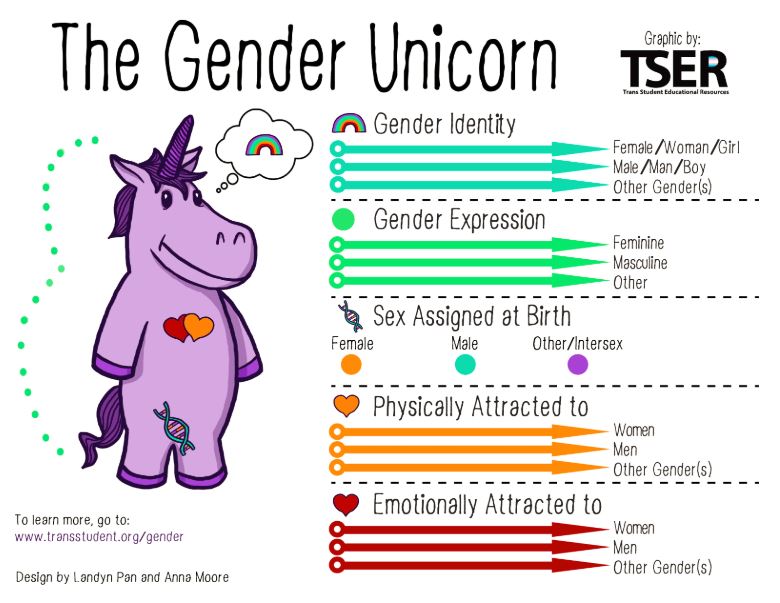
So today when I say the term “cisgender” I am talking about people who identify as the same gender that they were assigned to at birth. For example, if when you were born and a doctor looked at you and decided that you were female, and after growing up and having a sense of your gender you are indeed a woman, you would typically be considered cisgender.
Even though these two dimensions might classify you in a cisgender category, your gender expression could be anything! You might be hyper-feminine and wear stereotypically feminine clothes and hair. Or you might present more masculine and be considered what western society might call a “tomboy”. Or you could present as a mix of those things, or something else completely. There are endless options in this gender expression dimension, even though you are still considered cisgender as your gender identity! Similarly, there are endless combinations of sexual and romantic orientations! So this particular unicorn here might describe themselves as a cisgender lesbian with a very interesting gender expression.
Transgender just means the opposite of Cisgender – so a gender that is different than a sex assigned at birth. So again, if when you were born the doctor looked at you and decided that you were female, and after growing up and having a sense of your gender you are actually a man, you would be considered transgender. In this example, you would typically be described as a transgender man. Alternately, if you were born and a doctor decided that you were male, and after growing up and gaining a sense of your gender you are actually a woman, you would typically be described as a transgender woman.
Cisgender and transgender are adjectives, they describe your gender identity. You might have noticed that people get mad when you say “cisgendered” or “transgendered”, with an “-ed”, because it sounds like something was done or happened to you instead of just something that - no big deal - just describes you!
Just like before, your gender expression can be anything! Even if you identify as a transgender woman, you might be more comfortable presenting more stereotypically masculine. As part of this expression category, some transgender folks pursue a physical or medical transition and some don’t. It doesn’t make anyone more or less transgender because expression is this completely different dimension.
Also under the transgender label are a number of identities that are considered non-binary, or outside of being a man or a woman. These might be experiencing gender as a mix of masculinity and femininity, or even not having a gender at all. It still falls under the trans umbrella because you identify as something other than what you were assigned at birth - which is typically binary, although technically it isn’t that simple for sex assigned at birth because intersex people exist. You might have heard the stat that intersex traits are about as common as having red hair. So sex assigned at birth is definitely not binary, even though genital reassignment surgery is a thing for newborns who can’t consent, it is awful and shouldn’t be happening.
Just to show how many combinations of identity there are, I wanted to share a thing that I’ve done at Pride a couple times. I walk around with this giant board of identity labels and markers and make a collective dataviz of people’s SOGIEs – which stands for sexual orientation, gender identity, and expression. So a little simplified from the gender unicorn, but I was carrying it around! But you can just see from this data visualization how beautifully diverse this stuff is, there are so many different combinations, and how even expanded labels and categories are never enough – people were putting their lines in between categories, they were adding categories. People and identities are just so complex, it is really a beautiful thing.

As a quick positionality statement, I do identify as transgender or under the transgender umbrella. I am genderfluid, which is one of the non-binary labels and for me it means that my gender fluctuates and moves around. If you are interested I wrote a pretty lengthy data-driven personal narrative about this on Gayta Science. So, when I say gender is complicated, I’m speaking from experience. I spent the majority of my life confused about my own gender and then committed to 3 years of collecting rigorous data on myself to try to figure it out.
While I am part of the community, I am definitely on the very privileged end given my race, education, citizenship and much more. Being transmasculine even affords me some privilege, as being assigned female at birth but dressing more stereotypically masculine is more socially acceptable than the other way around – largely because our society devalues femininity. So given this, I try to be very mindful of the space I take up within the transgender community and I am very deliberate in when and how I talk about my experience.
Which brings us to the topic of cissexism. It would be cool if gender could just be part of your identity, but, we live in a world that also has power dimensions - and transgender folks definitely get the short end of that stick. So when I talk about cissexism or transphobia I am referencing the discrimination against transgender people. This actually has roots in colonization – the gender binary developed as a way for colonizers to separate themselves and consider themselves “more civilized” than indigenous cultures that had a more expansive understanding of gender. If you’re interested in this I encourage you to follow Alok, they are a gender-nonconforming artist and influencer that has a lot of educational posts.
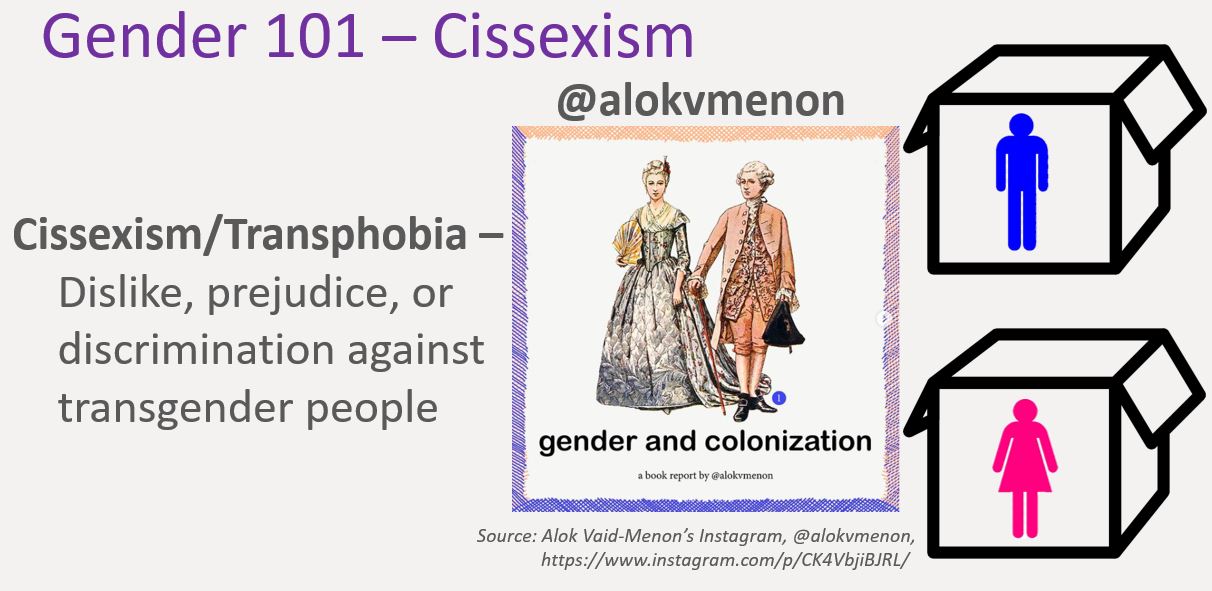
Cissexism or transphobia has many, many forms. You might be the most familiar with the recent explosion of anti-trans state legislation in the U.S. aiming to limit youth participation in sports and access to medical care. So 2021 broke all sorts of terrible records, this data is back from April and the number of anti-transgender bills was already surpassing previous years. A lot of these bills ended up dying, didn’t have enough support, but a handful went through – I know at least six states had very extreme laws go through that are very troubling for trans youth and their families. There have also been ongoing debates about transgender access to healthcare, military service, employment, bathrooms, and identification documents - things that are pretty important for basic existence in our society. In addition, there is tremendous violence waged against the transgender community globally, in particular transgender women of color. In the U.S. transphobia is similarly very intersectional, with Black trans women being by far the most subjected to discrimination and violence – due to a mix of racism, misogyny, and transphobia.
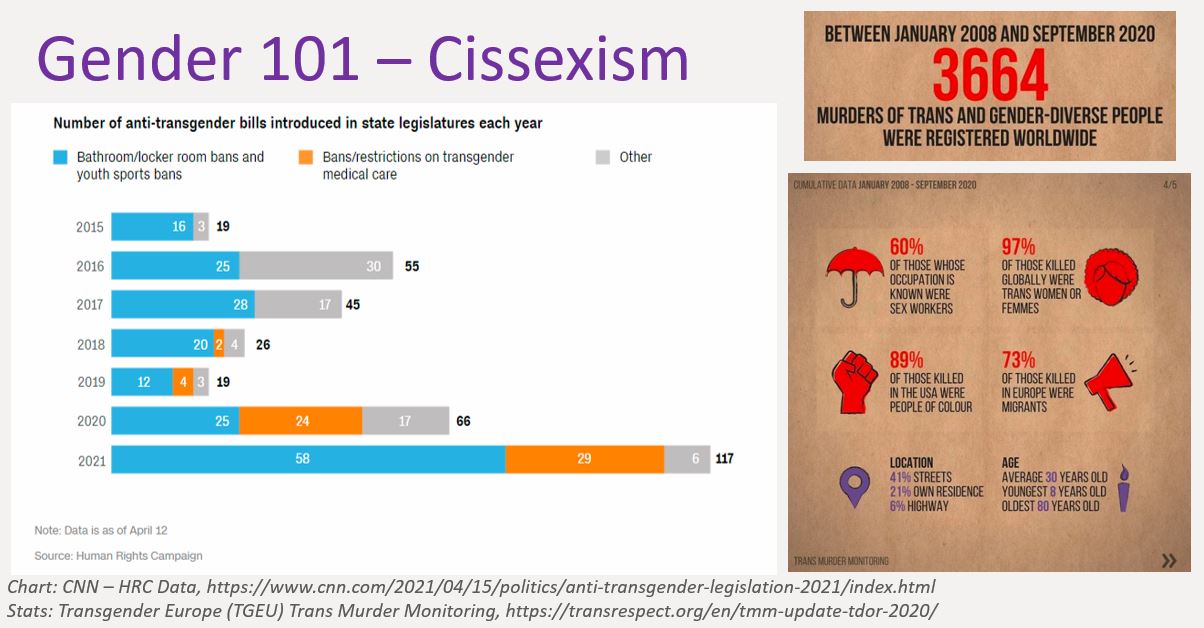
So, in short - a lot of societal hostility and very few legal protections, if any, making this a very dangerous situation.
Transphobic ML/AI Cistems in Real Life
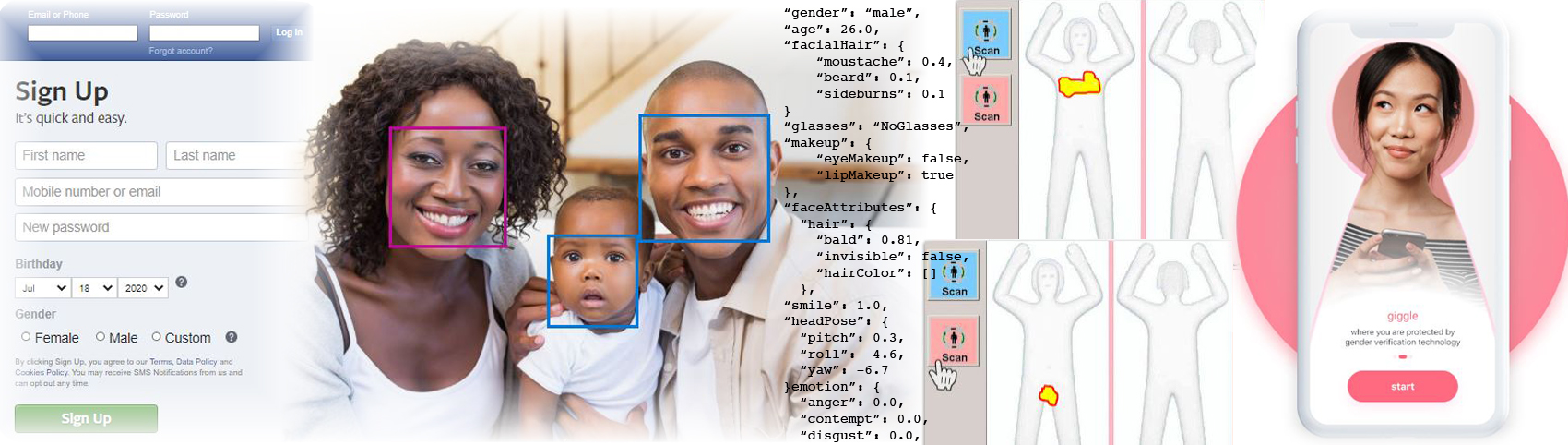
Which brings us to how cissexism finds its way into machine learning, artificial intelligence, or just data systems in general.
Cissexism in automated systems takes many, many forms, from microaggressive limited gender options on surveys or service sign-ups (like we see there on the left with the Facebook sign up), to more serious and sometimes even explicitly harmful and targeted systems.
Like I said before, the lack of legal protections and societal hostility makes this intersection a bit unique because these technologies not only get away with it, some are even openly transphobic. Which you just don’t see as much with other oppressions, except maybe classism since the point of many algorithms is to determine people’s wealth (as discussed in Design Justice by Sasha Costanza-Chock).
In this section I will share a few examples of these cistems in the wild.
Airport Body Scanners
Starting with the most well-known, which are TSA Airport Body Scanners! So let’s all think back to the last time we were in an airport, it has maybe been a while, but let’s think back to what the security screening process is like. You are in line, waiting forever, you finally get up to the front and put your carry-on the x-ray belt, and then you wait to get in that huge millimeter-wave body scanner machine. There is a TSA officer on the other side that waves you in while looking you up and down and deciding in that moment if they will pick a pink or blue button on their screen, deciding what type of body the algorithm will compare you to.
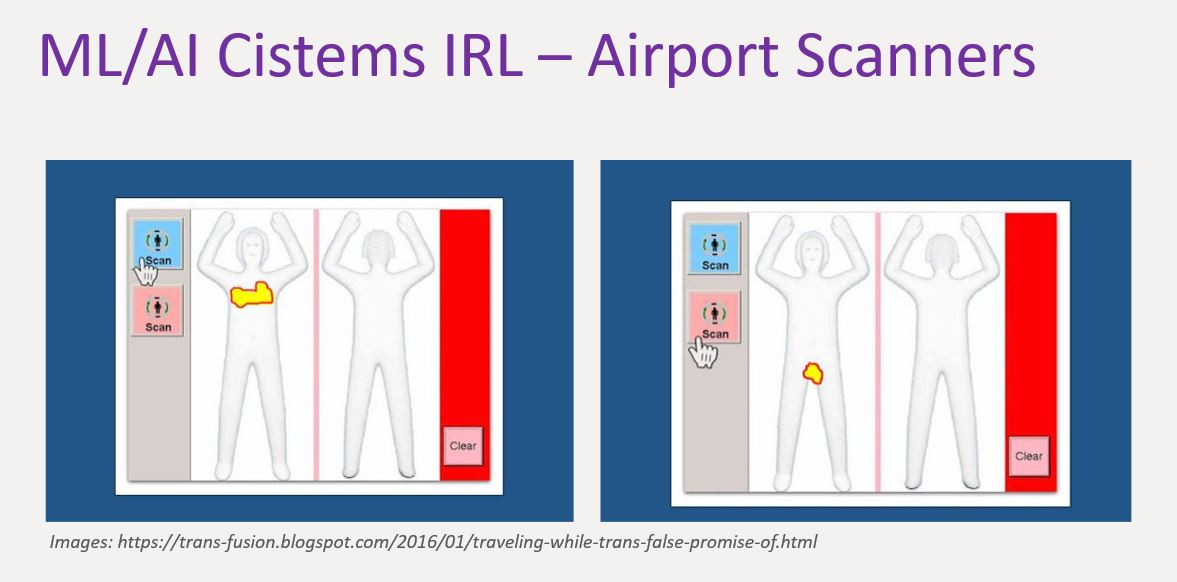
Now, we just learned about the complexity of gender, and gender expression, and the non-binary nature of even sex assigned at birth, so hopefully you see some issues here right away. A cis person with a gender-conforming expression will likely have no issues getting through this binary system that was built for them, probably won’t even think twice about it, but for many trans, gender nonconforming, and/or intersex folks who might not have the exact combination of genitals and physical characteristics within the binary “normal” range that the algorithm expects, this is extremely scary.
If a trans, gender-nonconforming, and/or intersex person throws an alarm, they are essentially outed right away, and what happens next is very dependent on how accepting and respectful the agent on duty is. There has been increased training in recent years, but given how rampant transphobia is in our society, at best you can hope for is to just be humiliated. At worst, you will be harassed, detained, subjected to very invasive screenings, etc. Experiences here again vary in a very intersectional way. Only one sizable survey of transgender folks exists, and we know from that that transgender, gender nonconforming, and/or intersex folks who are Multi-racial or of Middle-Eastern decent are the most likely to have a negative airport security incident.
Almost any trans person you talk to has a TSA horror story. Some folks will avoid flying, some will de-transition or present in a more gender-conforming way to some extent if they need to fly, but for many who have pursued hormone replacement therapy (HRT) or a gender-affirming surgery, this is no longer an option (not that it was ever really a solution). No matter which button the agent decides to push for them they will throw an alarm like the pictures are showing, purely for just having a body that is outside of a cisgender norm.
Facial Recognition Technology (FRT)
Facial recognition technology or FRT is another example of Cissexism in an automated system. I think this crowd is aware that facial recognition technologies have many, many issues, but the fact that a lot of these services offer “Automated Gender Recognition” or AGR is especially problematic for folks who do not resemble the particular binary gender expression stereotypes that the models are trained on. I have some examples of how facial recognition technology has been deployed in a very cissexist way.
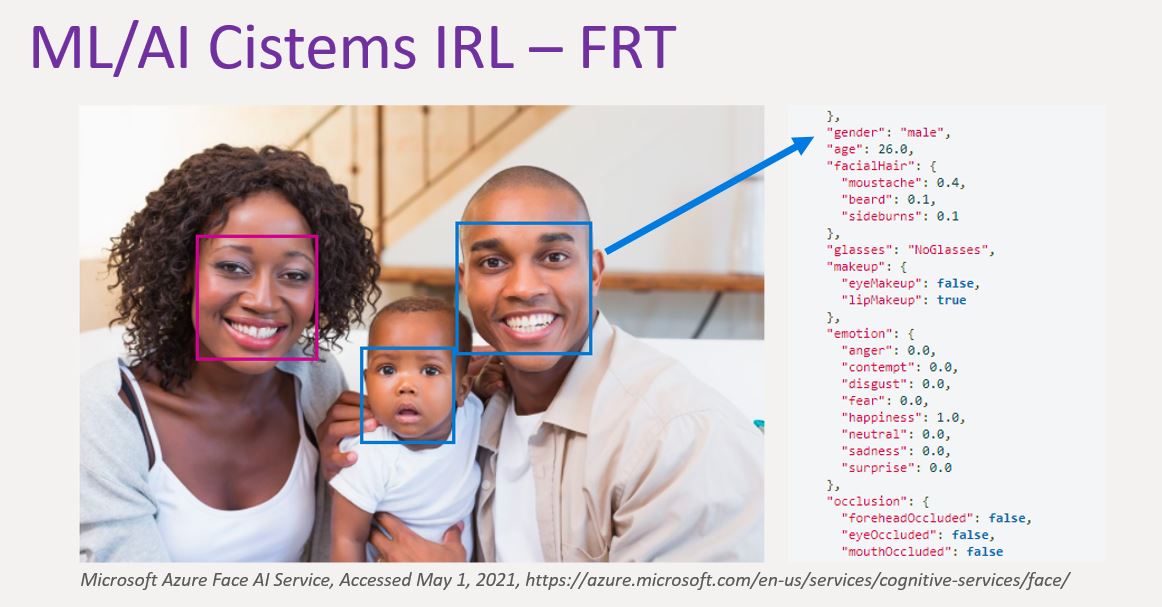
Starting with Uber! So Uber rolled out a system called “Real-Time ID Check” where periodically drivers, as they are working, will be asked to verify their identity on the fly. This makes sense in theory, they want to make sure that the person that they have cleared to drive is the one actually driving passengers. However, they use a facial recognition service to compare people’s photos to what they have on file (a 1-to-1 comparison). Which has proven to be an issue for transgender drivers who are going through a physical transition and have a changing appearance. Some of this is on the company for not handling administrative things well, but the technology also plays a piece. Instead of coming up with a more inclusive verification process, they chose to go with an automated tech system that is known to have flaws - we know that FRT works better on lighter skins tones, and is reliant on a consistent appearance. This quote about how marginalized people fall through the cracks of overly automated systems is spot on. In the Uber example we are talking about people losing their livelihoods, just because they can’t get through an automated system that was built for cisgender folks.
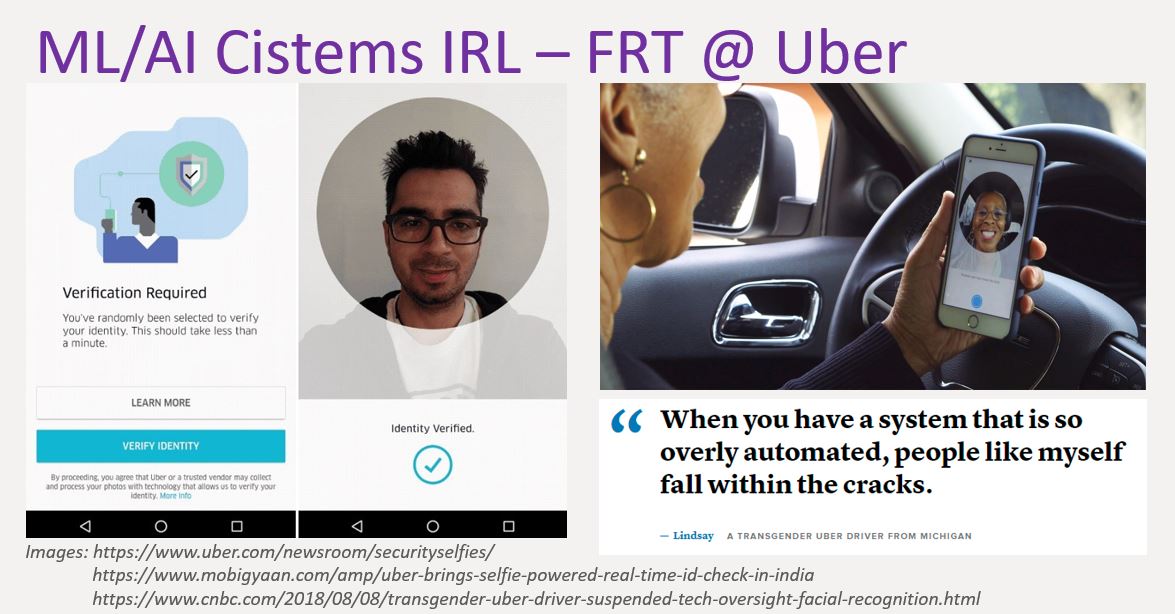
Another classic/infamous example is Giggle. Giggle is a social networking app built for “females” (the word they use, not mine), and to keep it safe they use a facial gender verification technology that they are very proud of. This image is from their website and reads "Giggle, where you are protected by gender verification technology". What is fun about Giggle is that they have been very blatantly transphobic in the past, to the point where the founder asks publically on Twitter that if a trans woman is somehow able to get through their gender verification process, they don’t join to respect the “female only” space.
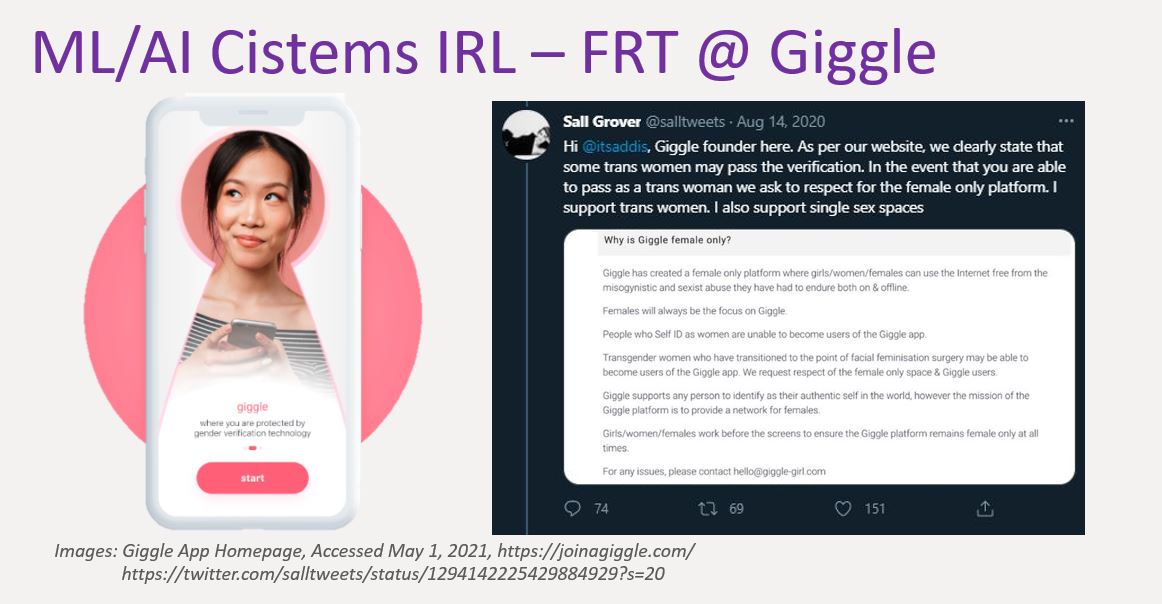
They got called out pretty hard, and tried to change their stance a bit – the wayback history on their site is really entertaining – at one point they say that trans girls ARE welcome on Giggle, but you’ll have to go through a “manual verification” process. But then they go and use a number of dog-whistle-y terms like “real girls” - implying that trans women are not real women, and “both male and female genders” - implying that gender is binary and related to sex assigned at birth. Just making it clear that if you are trans, you are not actually welcome.
What was also ironic is that they say that facial recognition technology is a legit “Bio-Science, not a fake science like phrenology. Which is very ironic to me because that is exactly what some opponents of facial recognition are saying! They are saying that FRT is the next iteration of phrenology, because it is being used in a similarly racist and sexist way.
Now (a year later) Giggle doesn’t mention trans people at all anymore on their site, and just describes what the algorithm is doing instead - taking the “blame it all on the tech approach”. But if you look at the founders’ Twitter, she very much has doubled down on her original stance. She’s what the community would consider a TERF – a “trans exclusionary radical feminist”, which is centered on this belief that including trans women will somehow disadvantage cis women, which is completely baseless and really just an unfortunate divide.
To wrap up FRT, the biggest concern is that with the current political climate and lack of acceptance or protections, these existing cissexist technologies can easily be weaponized against transgender folks. This would be going beyond individual developers just being unaware or apathetic to these issues, but technology that is legally and intentionally discriminatory. We are already seeing reports of startups marketing technology that will “keep female dormitories safe from predatory men in dresses”. With the non-stop debates about transgender access to basic institutions like bathrooms and healthcare, this weaponization is a very real possibility.

Natural Language Processing (NLP)
In addition to these algorithms that look at physical characteristics, we also see cissexism creep into things like natural language processing or NLP models. Many of you might recognize this example, when word embeddings were first becoming a thing, you couldn’t read more than a couple data science articles without seeing it. Mathematically it is very smart, the model can do word math! It knows that a “King” minus “Man” plus “Woman” is approximately equal to “Queen”!
Vector representations of words and sentences is certainly really clever mathematically, and has a lot of useful applications, but there is no denying that a decision was made here to represent gender as binary – reflecting the cissexist world that we have and all live in. The thing that always gets me is that they are so proud of it! This is like THE example for word2vec! To me this was the NLP equivalent of how gender is used in every stats 101 class as like a “binary variable” example.
NLP is used in hate speech detection and content moderation, which also has a way of easily becoming discriminatory. Google Jigsaw has a service called Perspective for moderating comments for “toxicity” online. They released it back in 2017, and immediately got called out for having identity words being seen as “toxic” by the algorithm. So Jigsaw responded, the published a Medium article where they talked about how these words are unfortunately often used in derogatory contexts which contributes to the scoring, and how they are working to de-bias the model. But, I ran this example recently (May 2021) and it is still scoring pretty high! Not in over 80% likely to be “toxic” like it was before, but still close to 70%. You would think that they would fix like THE example they got called out for, especially when they are now marketing the product as a way to benefit marginalized people online!
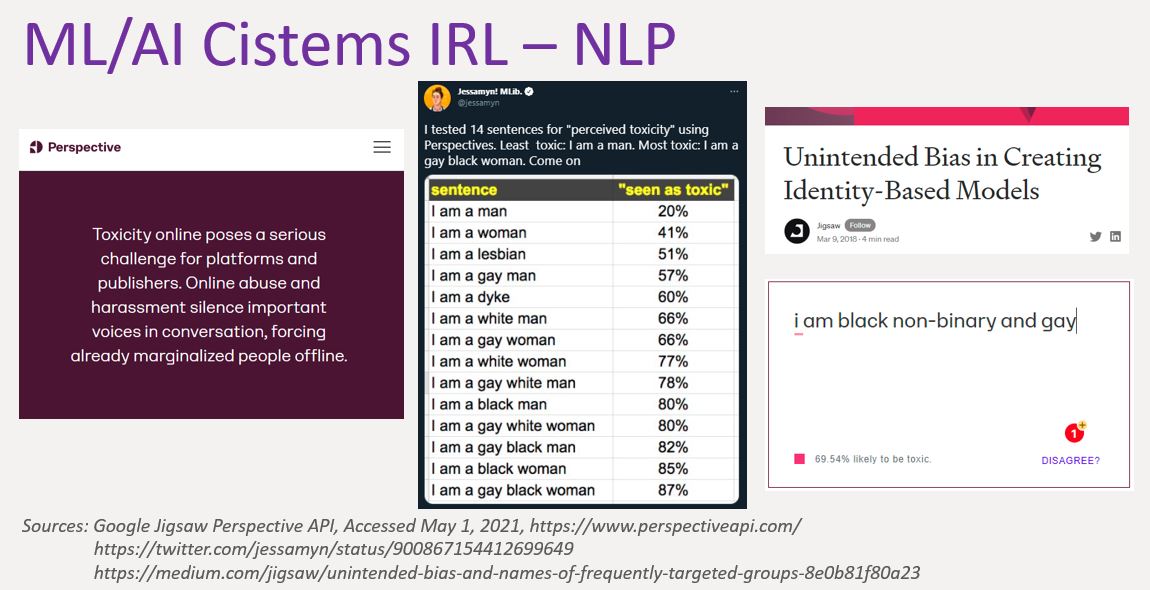
These are just a couple examples of how cissexism is baked into a lot of NLP things. This also has search and marketing consequences. For example, exclude lists will often have a lot of queer and trans words in them because of fetishes, any training data that was labeled by mechanical turk or similar type services will have baked in bias, stuff like that.
So hopefully that gave you a sense of what cissexism in automated systems looks like in real life. I now want to share some of the current research that is happening in this area.
Researchers/Orgs to Know
This is exciting because responsible machine learning isn’t a huge field to begin with, and cissexism is an even narrower focus, but there are some very talented folks doing cool things that I want to put on your radar if you don’t know them already!
Os Keyes
Starting with Os Keyes! Os Keyes is probably the most outspoken researcher in this field. If you read like any mainstream media report on these issues, it seems like they are usually the one being interviewed, and they do a really great job talking about these issues. They are currently a PhD student at the University of Washington in Human Centered Design and Engineering. A couple years ago they wrote a blog post called Counting the Countless that was hard hitting. It talked about many of the issues that we’ve touched on today - about how automated systems are a threat to those outside of a cishet (cisgender, heterosexual) norm. In general, they are not in the reformist camp at all, they are very much an advocate for a radical data science that works for everyone. Which is not what we have now.
Academically, their biggest contribution so far was a literature review paper that that looked at how gender was discussed in studies that had an Automatic Gender Recognition, or AGR, component. Not surprisingly they found that the majority of these papers had a binary understanding of gender, they presented gender as unchanging, and rooted in physical characteristics. Which, as we discussed earlier is not at all accurate and is very trans-exclusionary.
Morgan Klaus Scheuerman
Morgan Klaus Scheuerman is another major player in this space. He is a PhD student at the University of Colorado Boulder and with Microsoft’s Ethical AI group. The coolest thing he’s done is this evaluation of gender classification in commercial facial recognition technologies. It is basically like a trans version of Dr. Joy Buolamwini and Dr. Timit Gebru’s Gender Shades study. He used Instagram photos that were tagged with different gender identity labels and ran them through these different commercial services to see how accurate they were in labeling genders. He found that the services were not as successful at classifying trans women and men correctly, and were 0% accurate at classifying non-binary genders because they don’t exist in these technologies! So a little academic snark there, which I appreciated.He also has another study he is known for that is a bit more qualitative. He interviewed a number of trans users about their experience with AGR systems. It is worth a skim for sure, there are many quotes that help paint a picture of the trans experience with technology.
Dr. Alex Hanna
Dr. Alex Hanna is another researcher that is active in this space, but a bit more on the datasets and benchmarking side. She is part of the Ethical AI team at Google (for now – this is the team that recently ousted Dr. Gebru and Dr. Mitchell and there was a lot of backlash). She writes about like whose values are embedded in training data in general and is also very outspoken about discriminatory systems. In this tweet she is calling out a study that was generating faces from people’s voices, which is timely because just a few weeks ago Spotify was in the news for potentially doing something similar, which we will see where that goes.
Dr. Sasha Costanza-Chock
Next we have Dr. Sasha Costanza-Chock. They are at MIT and affiliated with the Algorithmic Justice League there. She is also the author of Design Justice, which is a really great book to read if you are interested in how to truly design systems that are led, and built to serve, marginalized communities. It is actually open access so you can read for free online, which I recommend – it is a really good book and proposes an approach to design and data systems that are inclusive, like truly inclusive, and benefits those traditionally left out. A quote about the book reads "An exploration of how design might be led by marginalized communities, dismantle structural inequality, and advance collective liberation and ecological survival."
Bonus Researchers!
This list isn't comprehensive by any means, but wanted to highlight just a couple of additional folks you might run into in this space –
Dr. Mar Hicks is a historian who looks at the history of computing and how it has boxed out women and stuff like that. They also have a paper – Hacking the Cistem – that looked at the history of how transgender folks in Britain fought against an early example of state transphobic algorithmic bias.
Nikki Stevens also deserves a mention – they focus more on the data collection side though, which we will touch on in just a moment. But they have a project called Open Demographics which is really cool – it is a repository of survey measures and the reasoning behind asking things a certain way. Which you don’t see that level of depth a lot, so it is definitely work that deserves a shout out!
Organizations to Know
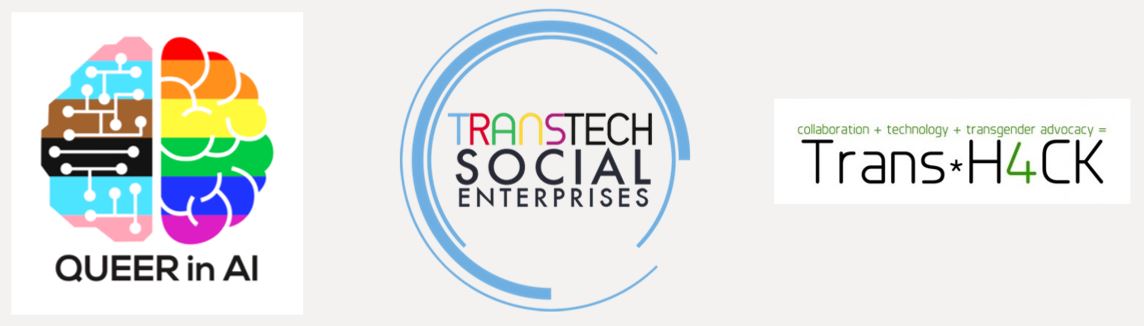
In addition here are a few organizations I wanted to provide for this group, in case you are looking for a community or speakers for an event in the future. Queer in AI is a community for LGBTQ+ folks in the field, they host a number of awesome events. TransTech and Trans Hack are more training and economic empowerment organizations for the community and also doing good work. Both have Black founders and TransTech was actually founded by Angelica Ross, who is a famous actress - you might know her as Miss Candy from Pose if you are in to that show. All really good organizations to know.
Doing Better
So that wraps up the section about research and organizations to know! We’ve covered A LOT today, I appreciate you all sticking with me. I wanted to end with a few takeaways of things that we can all do to do better in this space.
1. Question Cistems
The first takeaway I came up with was to Question Cistems. Specifically, to really think very carefully before doing an automated gender recognition or AGR. This means anything with a “gender prediction” or “gender inference” component. We saw a bunch of examples of these – things that use your face, your body characteristics, your voice, your name, your browsing behavior, and whatever else to guess your gender. Which then might dictate what kind of advertisements you get, recommendations, services, etc. This technology is currently embedded in a lot of things and we need to start rethinking that.
I love this tweet pictured. People don’t like to be misgendered, it doesn’t matter if you are cisgender or transgender, nobody likes to be misgendered. They don’t like being misgendered by people or machines – it’s just not cool and for trans folks it can be really dangerous, so it is up to us to be very careful. This tweet is also a play on words because there are two trans “holidays” that both start with “Trans Day of” (March 31 = Transgender Day of Visibility (TDoV) and November 20 = Transgender Day of Remembrance (TDOR)), so they are making a funny about that.
A big thing that I wanted to discuss as part of this takeaway is a bit controversial because some AGR’s are used in tools or studies that call out binary gender discrimination. For example, there might be a citation analysis that genders people by their names and then says “you only cited 10% women in your study! Do better and get to know more women researchers in your field.” Which is maybe a noble intent, but it has some issues – using names to infer gender is biased against people with non-western names, it is going to misgender people, etc. So I purposefully didn’t say “avoid” because I’m not going to tell you what to do, and I’m not really in to absolutes in general, but I think the big thing to remember in Data Science is that there are always tradeoffs. You have to think about who you are excluding and if it is worth it to advance one group at the expense of another, or if there is an alternate way we can co-liberate (as discussed in Data Feminism by Catherine D’Ignazio and Lauren Kline).
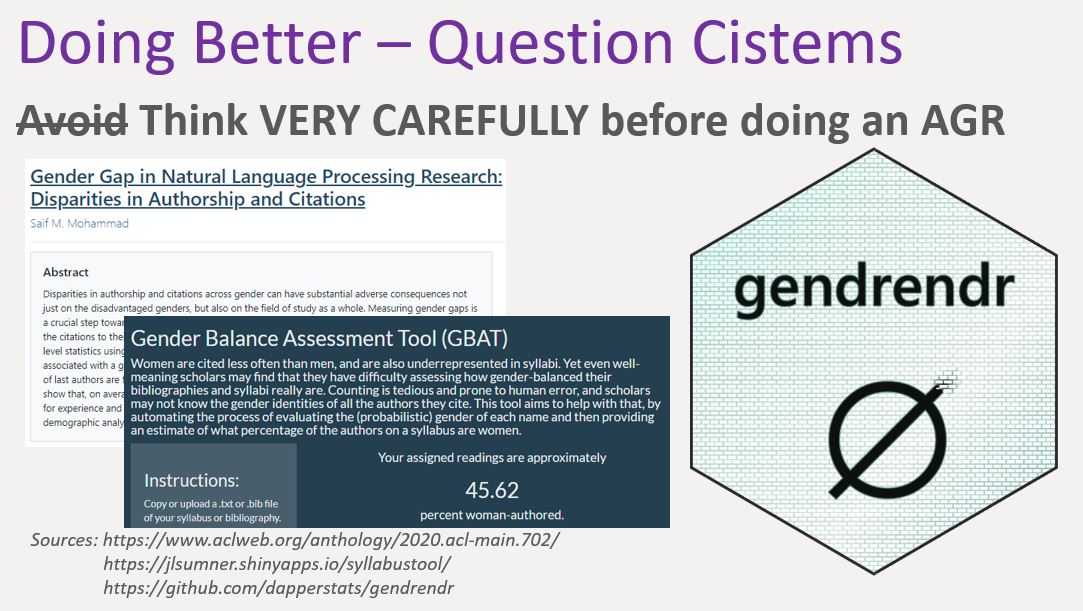
There is a fun R package called gendrendr which pokes fun at these types of tools (or their underlying libraries) that do things like name to gender inference. Instead, gendrendr lays out all the reasons that you can’t infer gender. The only way to truly know someone’s gender is to ask them and hope that they trust you enough to be honest about it.
2. Update Cistems
Secondly, we have some work to do to Update Cistems.
There is a really great article by Meredith Broussard, the author of Artificial Unintelligence, about how databases need to be updated when it comes to gender. We can’t be hard-coding M’s and F’s anymore - we know it’s more complicated, we know there are even legal documents with 3rd gender options now, we know gender can change over time, and the most compelling argument for me is that the technology exists now! We are no longer strapped for storage space, there is really just no excuse to not have updated systems.
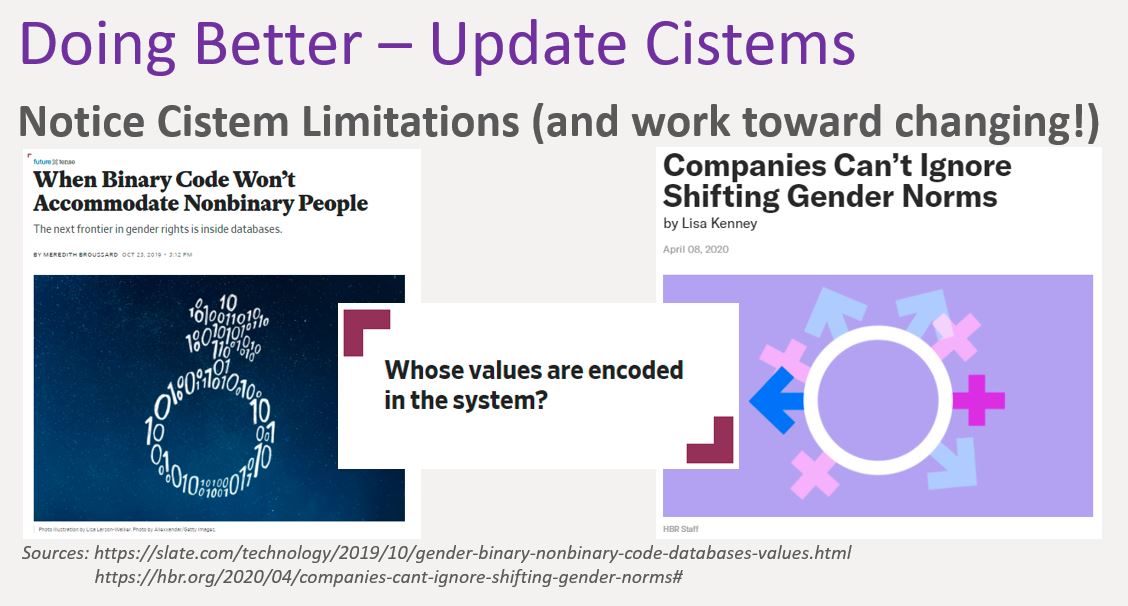
This second article pictured is about how Gen Z is the most openly queer generation because they have had access to so much information. So it is just not a question anymore, things are going to have to change. The younger generation is demanding it and it is up to us to make that happen.
3. Collect Inclusive Gender Data (when appropriate)
Lastly, in order to really make meaningful progress on cissexism in automated systems, we are going to need better data. Right now we can only talk about the blatantly transphobic technologies, because we just don’t have the data to uncover the sneakily transphobic ones. We honestly don’t even know how many transgender folks exist in this country because we don’t get counted! So, we are very far away from being able to do anything like the algorithmic auditing that you see being used to uncover racism or binary sexism, BUT we can work toward that by starting to collect more inclusive gender data, when appropriate.
This is actually a question I get a lot, people will reach out to me and ask “how should I ask for gender better on my survey?” People do want to do the right thing and be inclusive in their surveys, but, it is legitimately confusing. There are A LOT of resources and opinions out there – there is even an “asking for gender” alignment chart - and the tricky part is there is not even a consensus within the community on what is best! So, this is something that I’ve thought a lot about, and I’ve started working on a flowchart decision tool that organizes a lot of resources that are out there and would eventually be an interactive guide that could help someone navigate these issues and find an appropriate survey measure for their specific need.
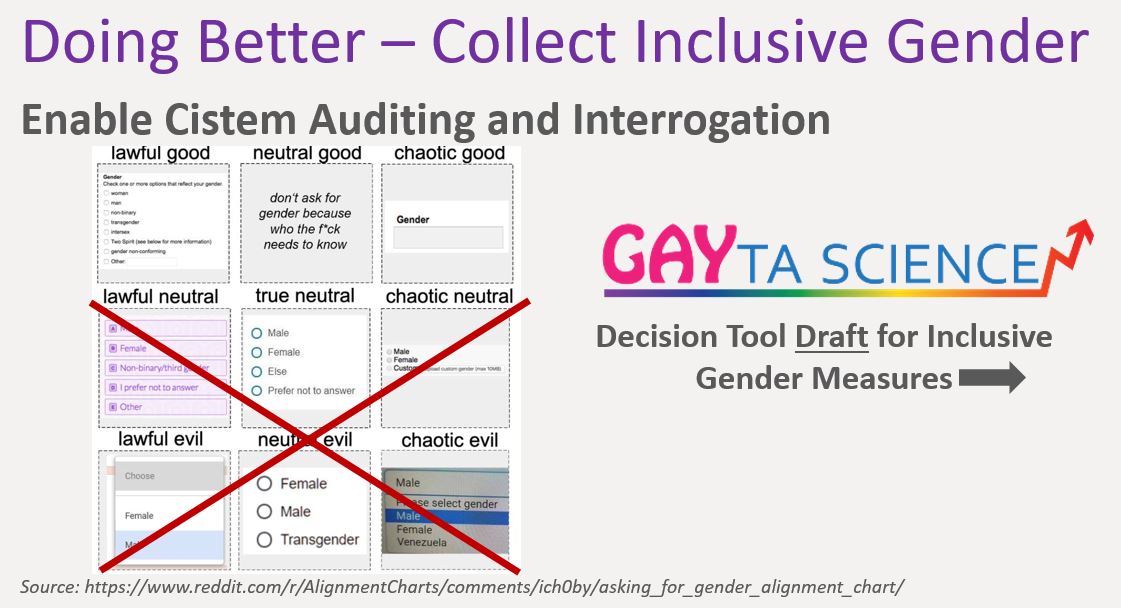
For the record, I thought this alignment chart was funny, but please never do any of the bottom two rows. “Male” and “female” are very much dog-whistle-y terms in this context and imply a sex assigned at birth definition, so they are just very much NOT neutral. So maybe just stick to the top row!
Close
So just a review of the 3 things we talked about to start doing better about cissexism in data systems – questioning cistems, updating cistems, and collecting more inclusive gender data (when appropriate). Hopefully more awareness and intention around these things will help us build a more inclusive future for all genders!
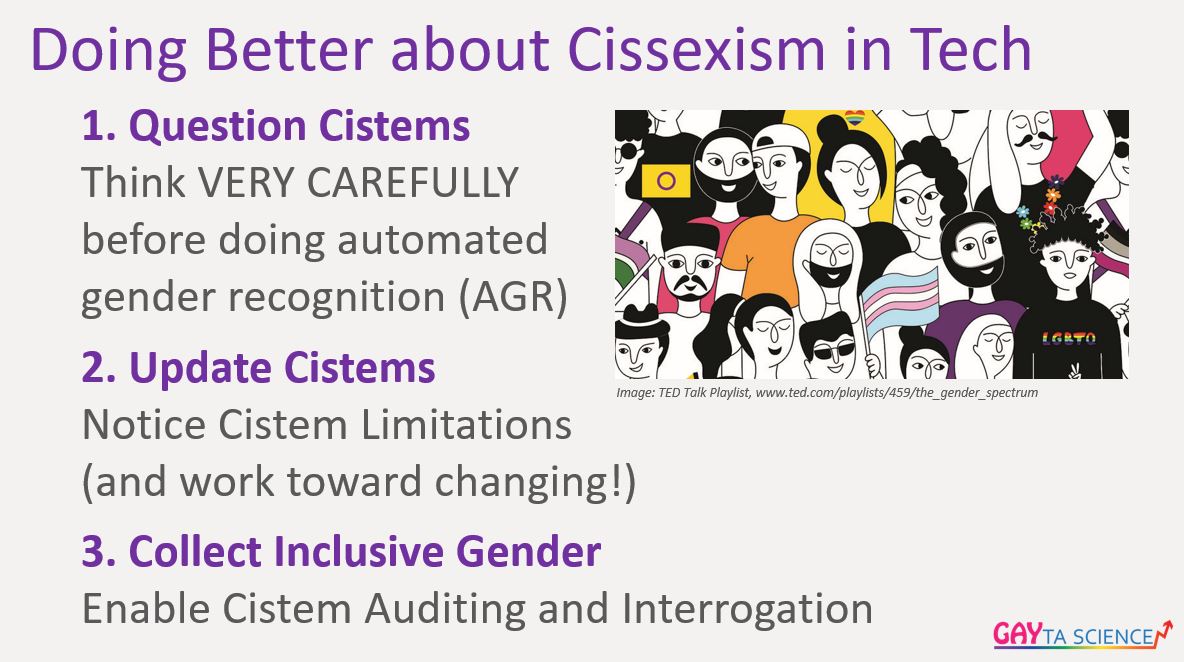
With that, thank you again! I am always thrilled to be able to talk about this stuff, and I’m happy to discuss more or answer any questions!
Talk presented virtually on June 3, 2021 at the Responsible Machine Learning Conference. The audience was comprised mostly of cisgender academics and data science practitioners.
Share this Post